Eye-tracking is a natural part of neuromarketing studies, and many companies specialize in using this as their main method. Recent reports suggest that in 2020, half of all online activity happens on the phone. This has led consumer researchers and market researchers to ramp up their focus on understanding communication on phones since it is known that users respond very differently on phones relative to PCs. But as we’re studying visual attention on phones, are we using the tools right? In a recent study, we demonstrate that optimized settings for your eye-tracking can improve accuracy by almost 20%.
The rise of phone attention
Eye-tracking has been used for more than three decades in studying different types of consumer behaviors. A quick search for the terms "eye-tracking" and "advertising" shows that this was an active field of research already in the 1990s, and as we enter 2021, we can estimate that around 10,000 articles are published on this each year!
However, the use of eye-tracking methods has traditionally been based on highly controlled environments. In the same period, the use of phones has increased dramatically. For example, in the US, the proportion of people who own a smartphone has risen from 35% in 2011 to over 79% in 2019. Naturally, there has been a dramatic increase in studies using eye-tracking to understand visual attention on phones.
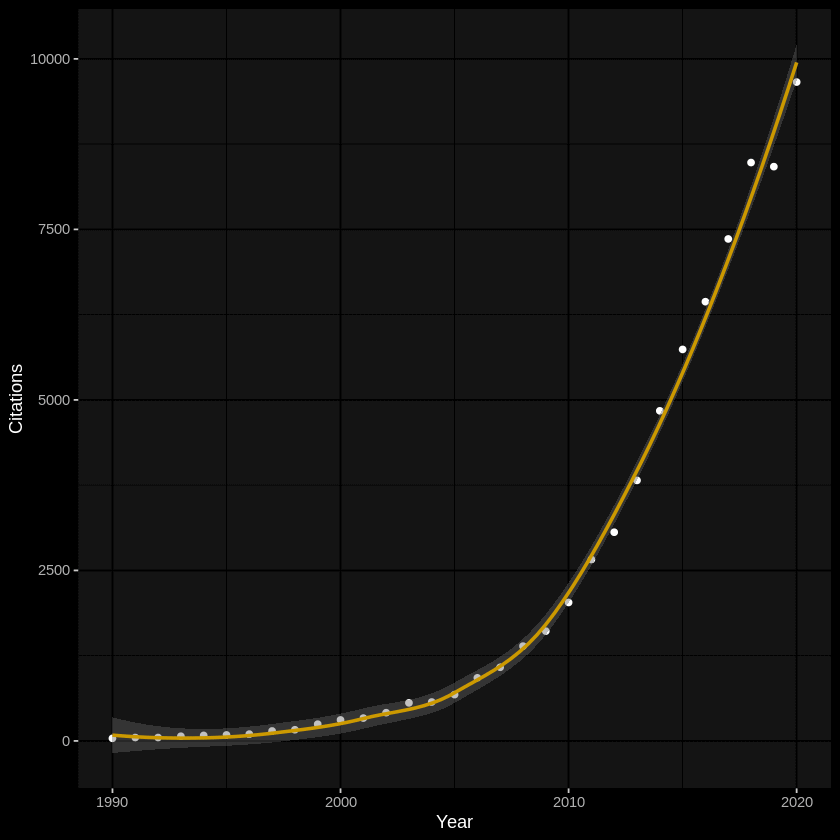
Measuring attention on phones
But there is much to suggest that attention on phones is a different beast than what eye-tracking was originally developed for. Even the world's largest provider of eye-tracking solutions, Tobii, suggests that you should not take standard settings for granted when doing eye-tracking studies on phones or other situations. More specifically, it means that the standard filters and settings that come with Tobii have been developed for certain use cases such as controlled lab settings with stationary eye-trackers. So if you are using eye-tracking glasses in your research, chances are that you are using the Tobii filters in a suboptimal manner.
Another aspect where phones differ from static environments is that the content itself is different. When you're scrolling the contents of your phone, whether it is a website or a social media feed, you are often following the contents with your eyes. This is something that is often referred to as smooth pursuit. The problem with smooth pursuit is that even though you are looking at the same item (e.g., a brand) as it moves across the screen, the eye-tracking system can see this as if you are looking at different places. After all, your eyes are moving across the screen.
Standard eye-tracking methods are not optimized for attention to contents on small screens.
With eye-tracking glasses, there is an additional challenge. Since you are moving your head, it can be difficult to track whether recorded eye-movements are due to the head moving or the eyes moving to compensate for this. For example, try looking at this dot ♦ while you are moving your head from side to side. Indeed, your eyes are moving right and left to compensate for head movement. So there are challenges ahead when using eye-tracking on phones, and a solution is needed. Adjusting the filter settings on things such as whether to merge adjacent gaze points is critical because it determines what is characterized as a single fixation or not. Basically, this will affect what you will conclude that viewers see and what they miss. This is illustrated in the figure below:
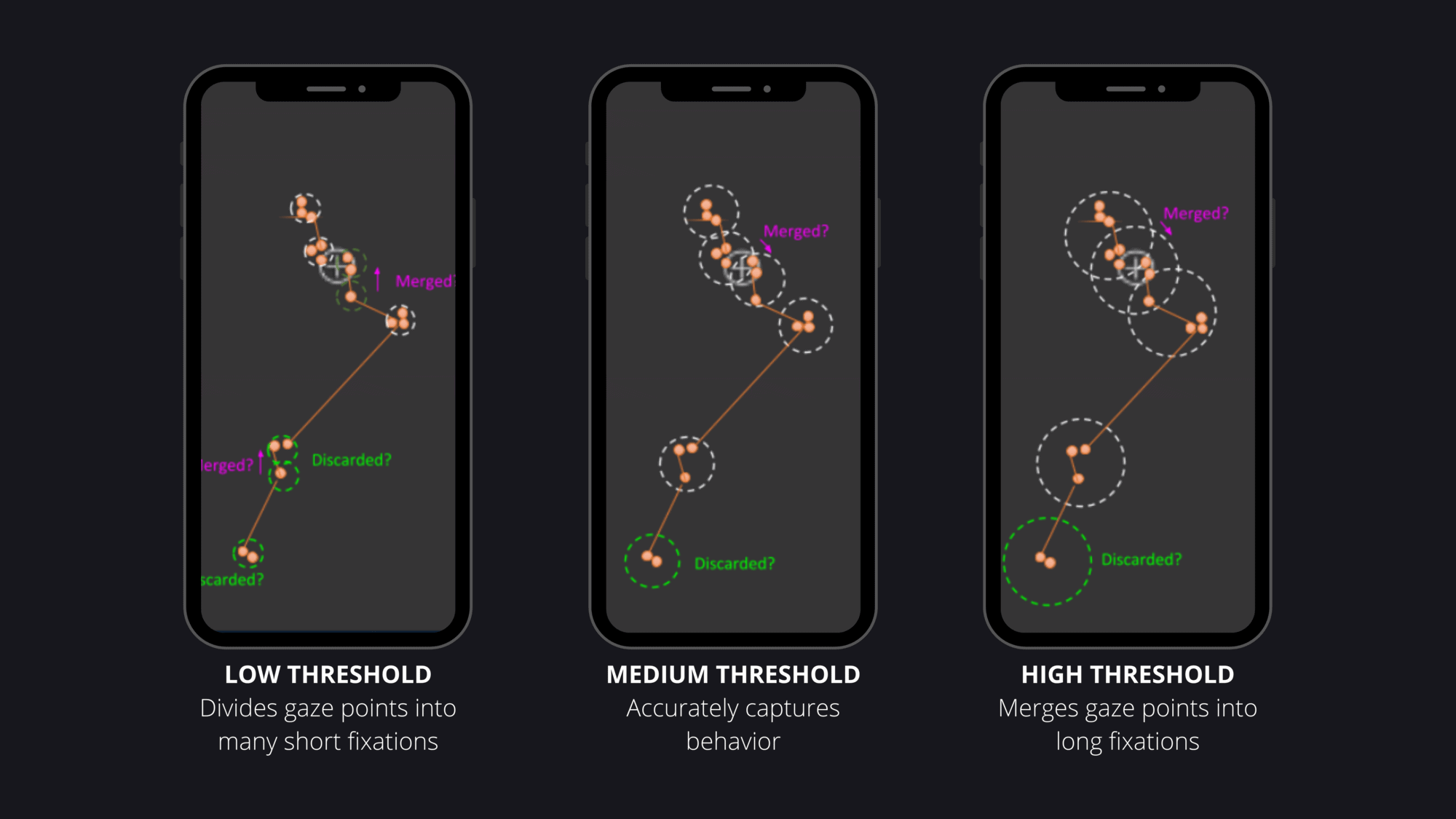
A Creative Commons solution
Now, we see the fruits of some intense work with some of the world's largest companies in social media and others. In a so-called "defensive publication," we document how it is possible to improve accuracy on eye-tracking for phones in a feed-based scenario. The methods we suggest improves accuracy with almost 20% compared to the standard Tobii filter settings. Put simply, the defensive publication via Creative Commons is to ensure that this is a free-for-all solution that cannot be patented. This means it is intended to make the industry better as a whole. The scientific manuscript is currently under review, and we hope that it will be published soon.
An improved attention metric
In our work, we focused on testing out a large set of different filter settings. We looked both at data where we had full control over the stimulus (e.g., a circle either statically changing position or moving across the screen) and where we had a high reliance on actual fixation and then tested in natural browsing behaviors. Below is an example of how we used different characteristics such as merging (yes/no), so-called discarding (yes/no), and then different types of velocity settings, meaning basically how much the eyes must move over time to count as a new fixation. In the graph below, please note the following concepts and goals:
- True positives (blue) indicate that when the filter says that it's a fixation, it really is a fixation -- this score should be as HIGH as possible
- True negatives (red) is a score when the fixation filter suggests that there is no fixation, and that this is correct -- this score should be as HIGH as possible
- False positive (black) is a score for when the filter setting says that it's a fixation when there really is not -- this should be as LOW as possible
- False negative (orange) is a score when the filter setting suggests that it is not a fixation when there really is -- this should also be as LOW as possible
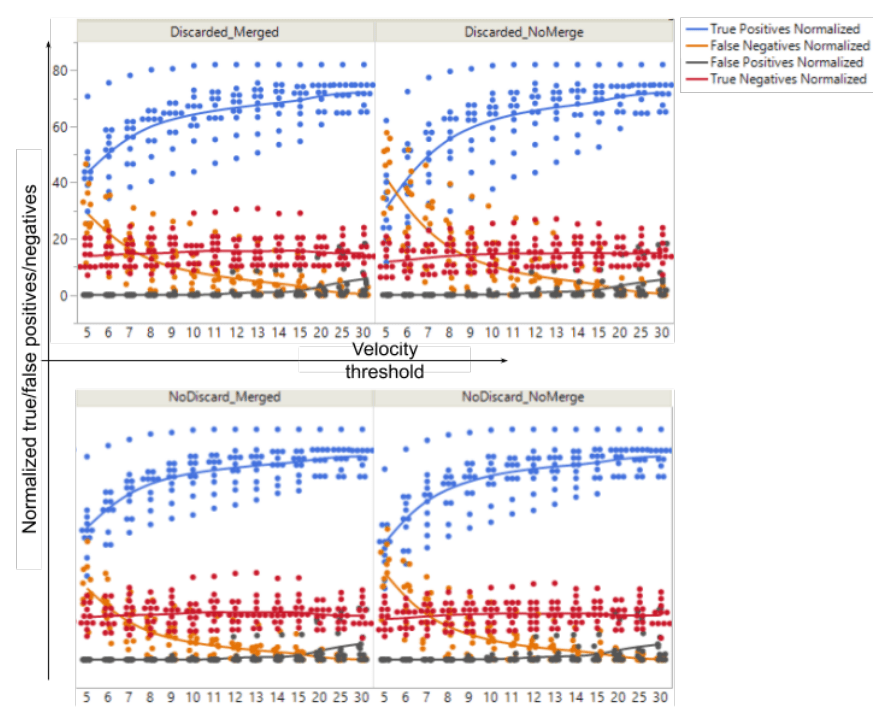
In this work, we identified the following setting to produce an optimal accuracy: using no discarding, adjacent fixations merged, and a velocity threshold of 11 degrees/sec. With this setting, we achieved a level of 93% on true positives while reducing false positives by 99.6%. The difference between the Tobii filter and the new, optimized filter, can be seen below on the same eye-tracking recording. As this movie shows, the optimized filter (right side) is much more sensitive and responds more to eye-movements on the screen.
Compared to this, the original Tobii filter (left side) is more static, and under-represents eye-fixations in the data.What this basically means, is that the original Tobii filter has too many false negatives and too low levels of true positives. Put another way, on phone-based browsing on social media and web pages, using the settings that Tobii glasses come with will lead you to miss fixations when there really are fixations. You will think that, for example, customers are missing the brand on a social media post, when they actually see it.
There's more work to be done!
In conclusion, this defensive publication offers every researcher that uses eye-tracking a more precise way to measure visual attention, as it is studied in consumer neuroscience, neuromarketing, and beyond. Beyond this, we also provide a framework for conceptualizing, measuring, and comparing different filter settings. This can be applied for any novel type of environment. This also ties in with my previous publication on a framework for increasing the validity and reliability of neuromarketing methods. What is coming up now is a series of publications from Neurons on further optimization and validation of eye-tracking on phones. Stay tuned!